Google Health’s human-centred observational study on AI’s real-world problems
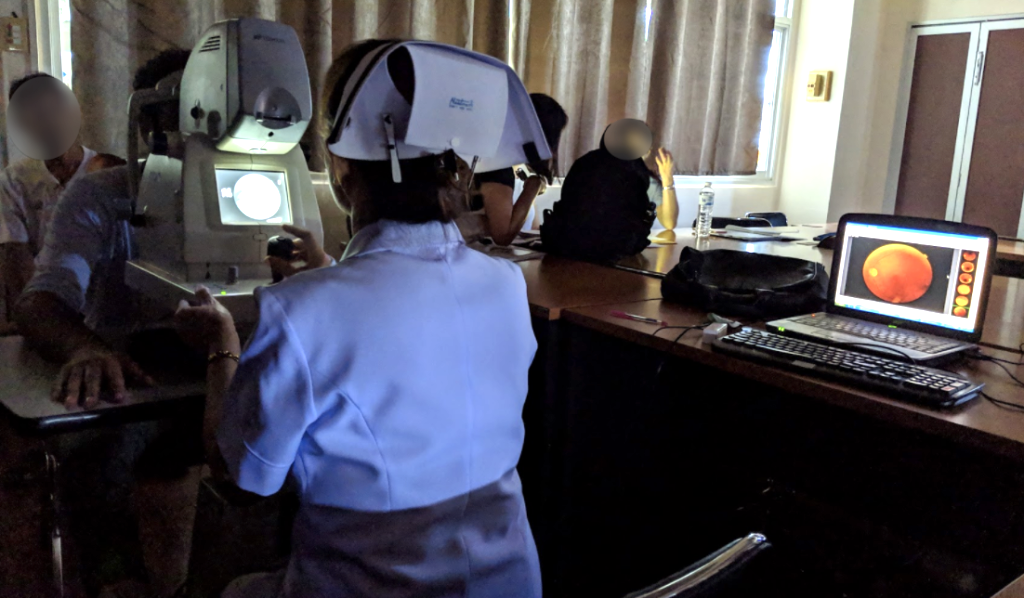
A Human-Centered Evaluation of a Deep Learning System Deployed in Clinics for the Detection of Diabetic Retinopathy
By Emma Beede, Elizabeth Elliott Baylor, Fred Hersch, Google, Anna Iurchenko, Lauren Wilcox and Laura Vardoulakis of Google, and Paisan Ruamviboonsuk of the Rajavithi Hospital, Thailand
CHI ’20: Proceedings of the 2020 CHI Conference on Human Factors in Computing Systems
April 2020 – Pages 1-12
Deep learning algorithms promise to improve clinician workflows and patient outcomes. However, these gains have yet to be fully demonstrated in real world clinical settings. In this paper, we describe a human-centered study of a deep learning system used in clinics for the detection of diabetic eye disease. From interviews and observation across eleven clinics in Thailand, we characterize current eye-screening workflows, user expectations for an AI-assisted screening process, and post-deployment experiences. Our findings indicate that several socio-environmental factors impact model performance, nursing workflows, and the patient experience. We draw on these findings to reflect on the value of conducting human-centered evaluative research alongside prospective evaluations of model accuracy.
> Presentation video
> Article in Financial Times
> Article in MIT Technology Review