Great engine, but the fuel seems poor. Discussing insight development in corporate marketing
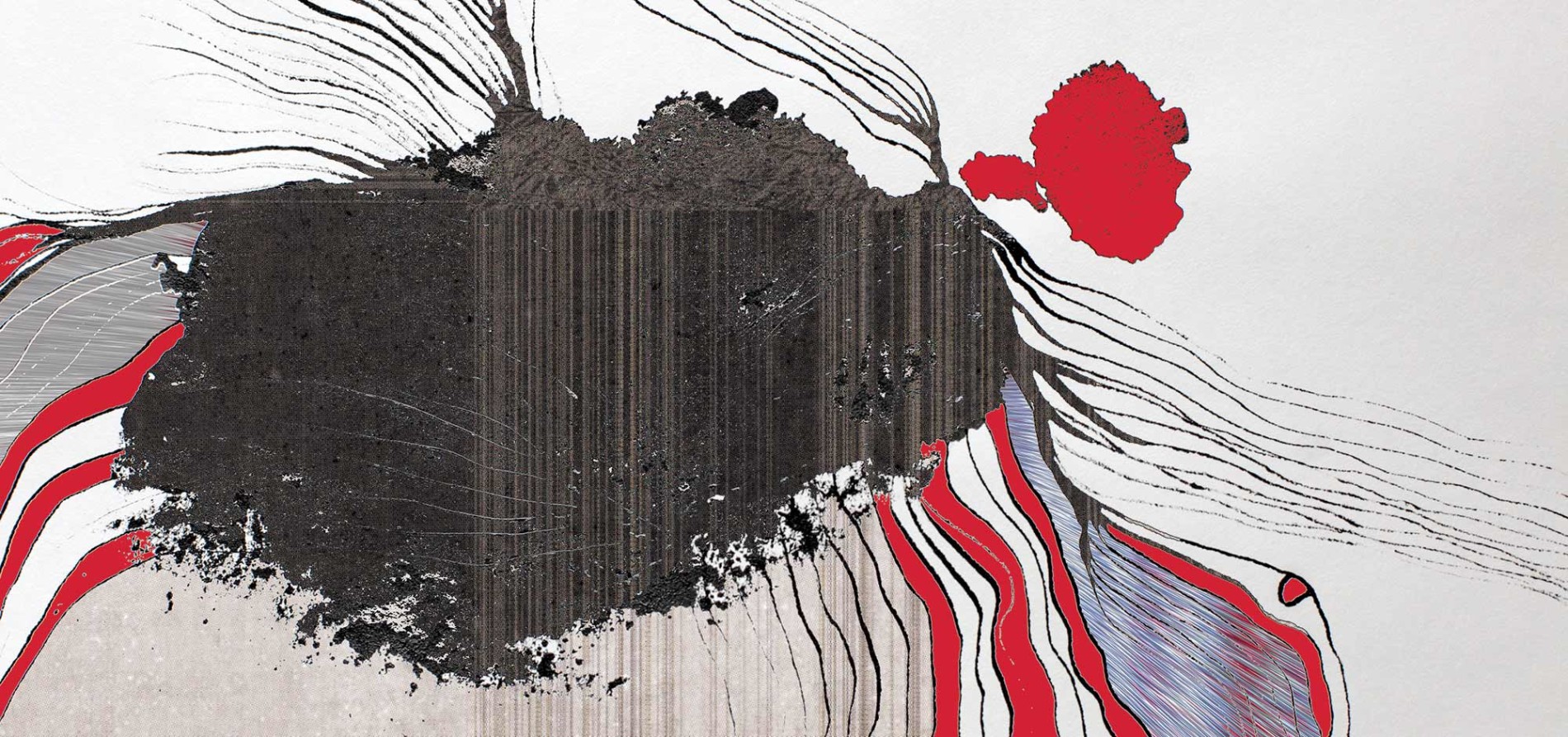
The September issue of the Harvard Business Review (HBR) contains a lengthy essay, entitled Building an Insights Engine, on how Unilever has created the organizational capabilities to “transform data into insights about consumers’ motivations and to turn those insights into strategy.”
The article was written by Frank van den Driest and Keith Weed of a brand and marketing strategy agency that conducted an extensive “customer centricity” industry survey for Unilever and Stan Sthanunathan, an Executive VP at Unilever.
Although the transversal and integrated organizational and operational capabilities are clearly advanced and makes the article more than worthwhile (we don’t think it’s an infomercial like one of the commentors), and its ethical anchoring is clearly sincere (much appreciated!), the underlying motivational model of human behavior, put forth in the HBR essay and the cited insights2020 white paper, seems instead quite outdated.
The goal is still “to distill insights about consumers’ motivations and needs on the basis of their behaviorâ€, Insight gathering is largely conducted through market analysis and data mining, some quantitative testing (e.g. facial expression testing), and very rudimentary qualitative approaches (e.g. the Discuss.io tool to arrange virtual meetings with consumers anywhere, real time consumer monitoring through Google Glass-like wearables, or managers wearing old-age simulation equipment “to bring the experience of older consumers to lifeâ€). It is in the end overlaid with a creative agency “whole brain†approach: “the capability to combine the science and analytics of what the data says (left-brain orientation) with the art of deriving creative recommendations (right-brain orientation) that resonate both internally and externallyâ€.
Actual observation of people’s behaviors, where Unilever staff “will go to people’s homes to wash clothes or cook a meal, seeing first-hand how users engage with Unilever products”, is described as a creative workshop exercise, rather than a real insight gathering method.
A more contemporary and methodologically diverse approach to obtaining human behavioral insights – inspired by behavioral economics and cultural change approaches and grounded in qualitative research – applies another conceptual framework altogether. It is centered around people’s concrete lives anchored in specific contexts with all their messiness, and focuses on their “goals” (instead of “needs”) and “behavioral patterns” (instead of “motivations”) *. The cultural and cognitive constraints of people’s behaviors are then further articulated through representations of cognitive biases, cultural frames and architectures of choices (to name but a few).
The means of applying such insights inside a corporation are then also very different. It is now no longer just about problem solving and analysis but instead about the types of mapping and modeling that can be derived from these goals, patterns, frames and architectures of choices.
In advanced UX agencies (such as Experientia) and some corporations this is done by conducting opportunity mapping and modelling to explore also unexpected and more innovative directions than those that a mere problem solving approach (where the problem is already defined) could lead to.
They conduct behavioral analysis and persona modelling that go far beyond the typical socio-demographic and motivational analyses, or technology-intensive data mining of consumption patterns. Such behavioral modeling approaches incorporate narratives that people actually use in their real lives to frame – implicitly – their decisions and to justify – more explicitly – their behaviors when given a selection of choices. They also help to explain the “why” of observed behavior, allow research and business teams to define the distinguishing factors that drive people’s behaviors, and suggest insights that enables this diversity to be transformed in choice architectures or product/service solutions.
While quantitative data can provide important signs and clues, often expressed through statistical correlations, they do not provide insights. The HBR article repeatedly confounds correlations with insights, and quotes some highly successful examples (e.g. the cases of “It Takes a Village†and “Love at First Tasteâ€), but there are many industry cases of correlations that have not been productive for business and were simply dead ends (e.g. because the two phenomena where both driven by a further – and not understood – third factor). Other “insights†are so sweepingly general (and brand-friendly) that they can hardly be derived from data mining: “Customers expect much more than good products or services: they expect a seamless, consistent, tailored-to-their-needs brand experience that goes beyond functional benefits and is built on a clear purpose for why the brand exists.†(from the Insights 2020 white paper that the article refers to). Real actionable insights require a deeper understanding of people’s narratives. These qualitative data can then be validated by quantitative analysis and data modeling and this integrated qualitative/quantitative validation will provide new strategies to nudge behavioral change.
In Experientia’s case, this all driven by a fundamental humanistic ethical stance aimed at helping people to make better decisions and responsible choices whenever possible.
Marketing agencies and departments – in particular those dealing with FMCG’s – will have to find ways to apply these contemporary approaches in their business practices to become truly customer-centric and much more effective, rather than remaining at the level of the exploration of needs and motivations, and developing “problem solving” approaches only based on advanced data mining. In fact many corporate marketing and innovation departments are moving this way already.
—–
* The HBR article also uses the term “behavioral patterns” but they are really correlational clusters derived from automatic video mining and other technologies.