Sharp methodological critiques on current Big Data practices
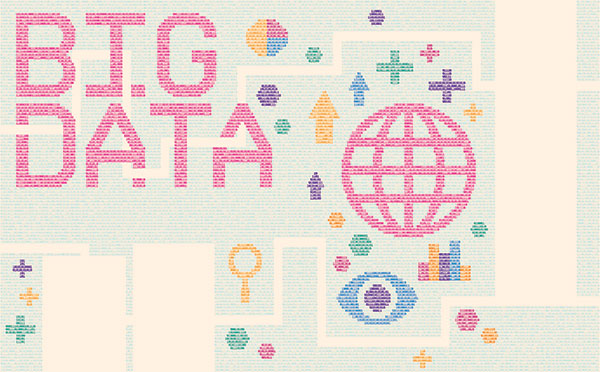
Two methodological critiques on Big Data that caught our attention:
In the Financial Times, economist and journalist Tim Harford points out that sampling bias and statistical errors are, if anything, magnified in Big Data research, and that theory-free analysis of mere correlations is inevitably fragile.
“Recall big data’s four articles of faith. Uncanny accuracy is easy to overrate if we simply ignore false positives, as with Target’s pregnancy predictor. The claim that causation has been “knocked off its pedestal†is fine if we are making predictions in a stable environment but not if the world is changing (as with Flu Trends) or if we ourselves hope to change it. The promise that “N = Allâ€, and therefore that sampling bias does not matter, is simply not true in most cases that count. As for the idea that “with enough data, the numbers speak for themselves†– that seems hopelessly naive in data sets where spurious patterns vastly outnumber genuine discoveries.
“Big data†has arrived, but big insights have not. The challenge now is to solve new problems and gain new answers – without making the same old statistical mistakes on a grander scale than ever.”
A more theoretical, but no less critical reflection emerges from an interview that Mark Carrigan conducted with Emma Uprichard (both University of Warwick sociologists) about the “methodological genocide” that occurs in many Big Data practices.
“My worry is that there are already modes of practice that are already being laid down, attitudes and cultures that are being normalised, laws being set up, and global networked infrastructures that are being created with little thought to given to what is ‘social’ about them – and indeed often by those with little or no training in how the social is considered to work. To many the question of what is ‘social’ about the data is not even a necessary question because there seems to be an assumption in some circles that the ‘social bit’ doesn’t really matter; instead what matters are the data. But in the case of big data, the data usually are the social bit! I cannot emphasis this point enough: most big data is *social* data. Yet the modes of analysis applied to big data tend to mirror approaches that have been long been rejected by social scientists. And importantly, they have been rejected not because of the ‘discomfort’ that comes from the idea of modelling human systems using modes of analysis useful to model and think about atoms, fluid dynamics, engine turbulence or social insects etc., although that may be part of it. These kinds of big data methodologies might well be used meaningfully to address certain kinds of questions and ones that we haven’t even asked before. But one of the main reason these ‘social physics’ approaches tend to be rejected by social scientists is that these methodological approaches don’t so much as even a nod to how important social meaning, context, history, culture, notions of agency or structure might be – and yet these matter enormously to how we use data to study social change and continuity.
At the end of the day, it doesn’t matter how much or how good our data is if the epistemological approach to modelling social systems is all backwards. To use big data to simply drive forward positivist notions of the social without accounting the important of history, culture, meaning, context, agency and structure in shaping social life is on a hiding to nowhere good. Yet so far the signs are precisely that big data analytics are going down that path. So when I say that there is a methodological genocide going on, what I am getting at is that at a methodological level, the data analytics need serious interrogation particularly in terms of exactly they can or are going to improve our lives. “